With the non-stop growth of Tesla, Waymo, and Cruise, self-driving cars have emerged as transformative technologies and have played an important role in the future of the automotive industry. Let’s explore how data annotation drives the self-driving car industry in this article.
As a part of this development, data annotation and labeling are crucial processes to identify and categorize data, enhancing the advancement of AI technologies and driving their decision-making capabilities.
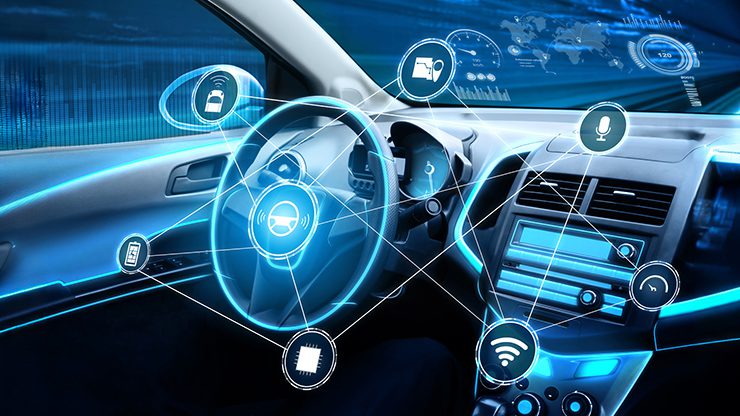
What is Data Annotation?
Data Annotation is part of the preprocessing stage including attributing, tagging, or labeling unstructured data when developing a machine learning (ML) model. This process is essential for training AI models to understand and classify the information.
Alongside machine assistance, the vital role of Humans [1] comes to the fore in the data annotation process.
The common data annotation types depend on specific use case requirements:
- Image Annotation: Mark objects and key points with bounding boxes, polygon, and semantic segmentation,…
- Video Annotation: annotating objects or actions within video sequences, enabling tasks such as action recognition, and object tracking.
- Audio Annotation: labeling audio recordings with speech recognition, speaker identification, and emotion detection.
- Text Annotation: Extract meaningful information from text documents.
The size of the global market for data annotation tools is expected to continue growing at a CAGR of 26.3% from 2024 to 2030)[2]. Due to the rise in the adaptation of AI within various industries, the demand for Data annotation services keeps rapidly evolving.
The Revolution Self-Driving Technology
A self-driving car also known as an autonomous vehicle is defined by the University of Michigan Center for Sustainable Systems[3] as ‘the technology to partially or entirely replace the human driver in navigating a vehicle from an origin to a destination while avoiding road hazards and responding to traffic conditions’.
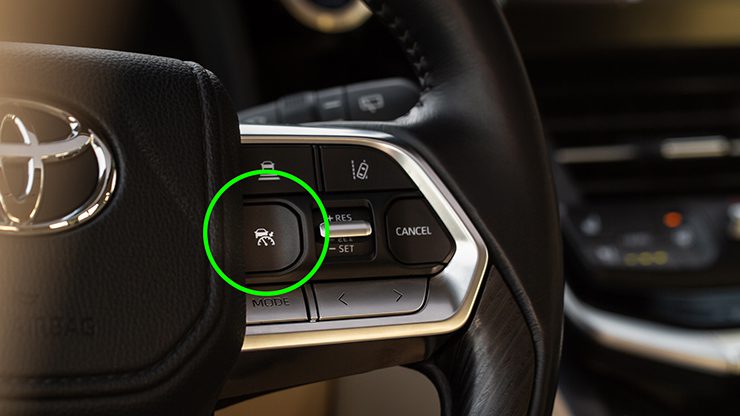
In 1998, Toyota brought Active Cruise Control (ACC) to the car industry. This allowed the car to keep a safe distance from other vehicles while maintaining a certain speed. The journey saw a significant uplift with the advent of modern computer technology in the late 20th century when Tesla launched Autopilot in its Model S sedan in 2015.
In 2017, Waymo launched the world’s first public self-driving ride-hailing service in Phoenix, Arizona. This meant people could hail a self-driving car without a human safety driver behind the wheel.
Today’s self-driving cars utilize a combination of forward-facing cameras and radar devices to gather information about road conditions for later analysis. Self-driving cars are not a far-fetched idea anymore; it’s already on the horizon.
Despite the upcoming challenges to developing self-driving cars, this new movement offers a new era of mobility, efficiency, and safety.
How Data Annotation Drives The Self-Driving Car Industry – The ‘Engine’ Behind the Wheel
For self-driving cars to be truly capable of driving without human involvement, an amount of training must be undertaken for Artificial Intelligence (AI). It will help self-driving cars examine what it’s seeing, and make the right decisions in every traffic situation.
But what’s behind the technology? The answer lies in How the data is annotated.
The Hidden Power of Raw Data:
Data serves as the ‘bedrock’ of self-driving car development. During this process, a large amount of data is continuously captured from sensors (LIDAR, camera, GPS, radar).
This provides abundant raw data about the vehicle’s surroundings and environment. These raw data are then processed to provide context and labels necessary for training sophisticated algorithms.
This process has become integral to algorithm training, elevating the robust models capable of navigating complex real-world scenarios with precision and safety.
What Kind of Annotated Objects for Self-Driving Cars?
Self-driving vehicles rely on the ability to understand their surroundings. This critical function is achieved through a complex process called object annotation.
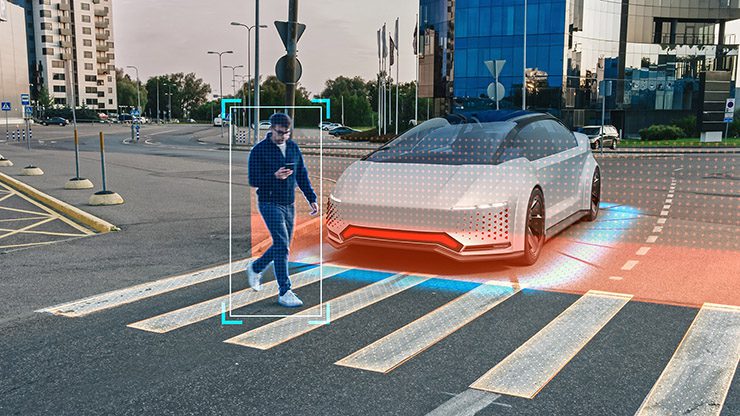
This annotated data serves as the training ground for the car’s artificial intelligence, enabling it to ‘see’ and interpret the world around it.
Here are some of the key annotated objects for self-driving cars:
- Vehicles: moving objects sharing the road, from cars, trucks, and motorcycles to bicycles and even horse-drawn carriages in specific regions. Allowing the self-driving car to accurately detect vehicles, maintain a safe distance, and predict their movements.
- Pedestrians including adults, children, and animals are accurately annotated to ensure the vehicle can detect them to avoid collisions.
- Traffic Control Devices: The car’s AI learns to recognize vital information on traffic signs, traffic lights, and lane markings, such as speed limits, stop signs, turn signals, and lane designations.
Data Annotation and Labeling Types:
Self-driving cars use a variety of data annotation approaches to label and annotate different aspects of sensor data. Some of the used data annotation and labeling methods are:
- Bounding boxes: are rectangular outlines drawn around items of interest, such as vehicles, pedestrians, bicycles, and barriers, to show their location and size within an image or frame of sensor data.
- Polygon Segmentation: Outlining items in photos with polygonal forms so that algorithms can distinguish between them and backgrounds.
- 3D Cuboids: This method involves sketching cuboids around items to help the system comprehend their dimensions, to better identify them in the future.
- Semantic segmentation: labeling each pixel in a picture with a corresponding class label, such as road, vehicle, pedestrian, or background, to offer information about the scene’s many items and regions.
Why Is Data Annotation and Labeling Important For Self-Driving Cars?
An important factor in autonomous vehicle training is the quality of the data annotations. More precise and thorough data annotation makes autonomous cars safer to employ in real-world situations.
This approach is necessary to guarantee that the machines receive properly labeled data for error-free decision-making.
Boosting the Vision of the Vehicle
The proper operation of autonomous driving depends on the annotation and labeling of data. To encourage safe driving, roadside items and components such as cars, pedestrians, barriers, and traffic signals must all have the proper labels.
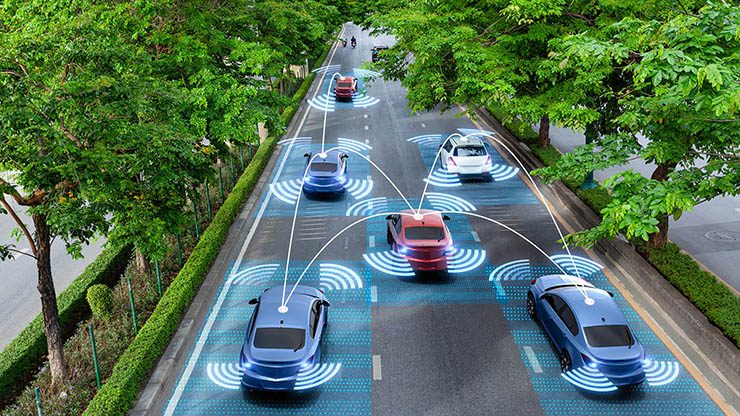
Integrated and annotated multi-frame sensor data from cameras, radar, LiDAR, and other sensors are used to develop robust sensory fusion algorithms.
Data annotation can also provide reliable semantic separation of the road surface, lane markers, and walkways.
This allows self-driving cars to stay in their lanes, calculate several routes, and select the optimal one.
Avoiding Unexpected Accidents
One of the most widespread benefits would come from increasing safety and reducing human error – which accounts for 90% of road accidents[4].
To ensure safety, large volumes of annotated sensor data were used to train AI models, which account for a sizable portion of this prediction power.
These models have the ability to track nearby vehicles, people, and objects, keep an eye on and assess the surroundings, and predict how the vehicles will move in the future.
The car could abruptly brake or shift lanes to prevent a possible collision. There might be serious consequences for this.
Effective Driving Scenarios Training
- Analyzing the Driving Scene:
Data annotation and labeling improve driver safety by assessing the driving environment and notifying the driver of potential risks.
The sensor data includes bounding boxes for people, cyclists, animals on the road, and other hazardous objects. These tagged photos are used to train algorithms to detect these potential threats.
Lane markers, road boundaries, and vehicle localizations are also marked to provide lane departure and blind spot alerts. These systems notify the driver if the car unintentionally drifts out of its lane or if another vehicle enters the blind area when changing lanes.
- Recognizing road signs and traffic lights:
To follow traffic rules and regulations, a self-driving car must immediately detect and understand road signs and traffic signals. Annotated photos of various traffic signs and lights serve as training material for AI models that enable this feature.
These models, trained on high-quality annotated data, can correctly identify stop signs, speed limit signs, yield signs, traffic lights, and other essential traffic signals.
This enables the autonomous vehicle to make intelligent judgments, such as stopping at a red light or altering its speed based on the speed limit.
How DIGI-TEXX Can Help You With Data Annotation and Labeling in Developing Autonomous Vehicles?
The journey towards self-driving cars hinges on one crucial element: high-quality data. At DIGI-TEXX, we understand the intricate world of autonomous vehicles and the massive amount of labeled data needed to train their AI systems.
Our data annotation and labeling solutions add value to any business from various industries: construction, insurance, automobile, etc.. around the world. We provide a team of specialized annotators with an experienced and scalable crowdsource, ensuring the delivery of high-quality training data.
With DIGI-TEXX’s data annotation service, we help you tackle complexity at scale by providing accurate tagging and labeling for both structured and unstructured data. We guarantee high-quality output and handle projects of any size or complexity.